Can We Trust Scientific Discoveries Made Using Machine Learning?
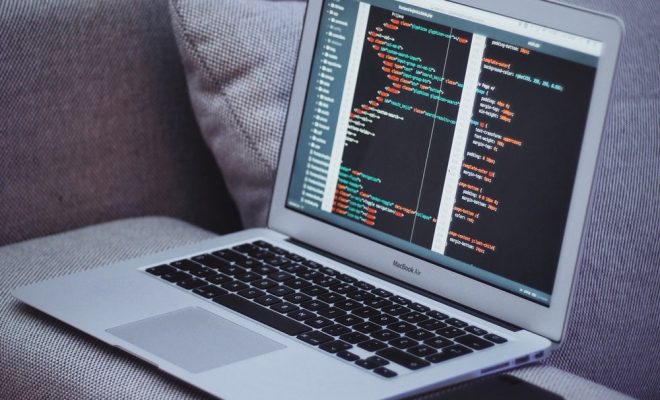
Artificial intelligence (AI) and Machine learning (ML) have accelerated scientific discoveries at a pace that would otherwise have been impossible. Machine Learning is an application of AI that provides a system with the ability to automatically learn and improve from experience without being explicitly programmed to do so. AI algorithms are widely used in scientific research. They are being applied to discover new drugs, to better understand the evolution of galaxies, to discover new chemical compounds and much more. The only problem is, can we trust the results that the algorithms come up with?
At least one statistician has cautioned the scientific community against this course of action. In a press release before the 2019 Annual Meeting of the American Association for the Advancement of Science (AAAS), Dr. Genevera Allen, associate professor at Rice University, told scientists to keep questioning the accuracy and reproducibility of scientific discoveries made by machine-learning techniques until researchers develop new computational systems that can critique themselves.
A scientific crisis
At the AAAS meeting she told scientists that the increased use of such systems was contributing to a “crisis in science”. She warned scientists that if they didn’t improve their techniques they would be wasting both time and money, reports the BBC.
Machine learning software is widely used to analyze data that has already been collected. ML depends on large data sets to discover useful patterns that can alert scientists to factors they were previously unaware of. ML is applied across many different subjects using existing data sets that are large and expensive.
Reproducibility crisis
Dr. Allen pointed out, however, that the results the software come up with are probably wrong and not to be trusted because the patterns that the software is identifying, exist only in the particular data set and not in the real world.
Allen added that often these studies are not found out to be inaccurate until there’s another really big dataset that someone applies these techniques to and then discovers that the results of the two studies don’t overlap.
Allen said there is a general recognition of a reproducibility crisis in science right now, and added: “I would venture to argue that a huge part of that does come from the use of machine learning techniques in science.”
Reproducibility is a hot topic in science at the moment and most scientists agree that there is a crisis. In a survey by Nature, over 70% of scientists revealed that they’d tried and failed to reproduce another group’s experiments.
The reproducibility crisis in science refers to the large number of research results that are not confirmed when another group of scientists repeats the same experiment. Which results should be trusted then? According to Allen, the situation is exacerbated by machine learning and big data systems.
Flawed patterns
Machine learning systems and the use of big data sets make the situation worse because the algorithms are written for a specific purpose: to look out for and find certain things in the data sets. So, when they search through huge amounts of data they will inevitably find a pattern, Allen told BBC News. She is questioning whether those results can really be trusted and whether they even represent scientific discovery.
The BBC reports that Dr. Allen is working with a group of biomedical researchers at Baylor College of Medicine in Houston to improve the reliability of their results. They are developing the next generation of machine learning and statistical techniques that can report on the certainty or uncertainty of their own results and whether the results could be reproduced or not.